Обработка естественного языка (NLP) в JavaScript (серия 2)
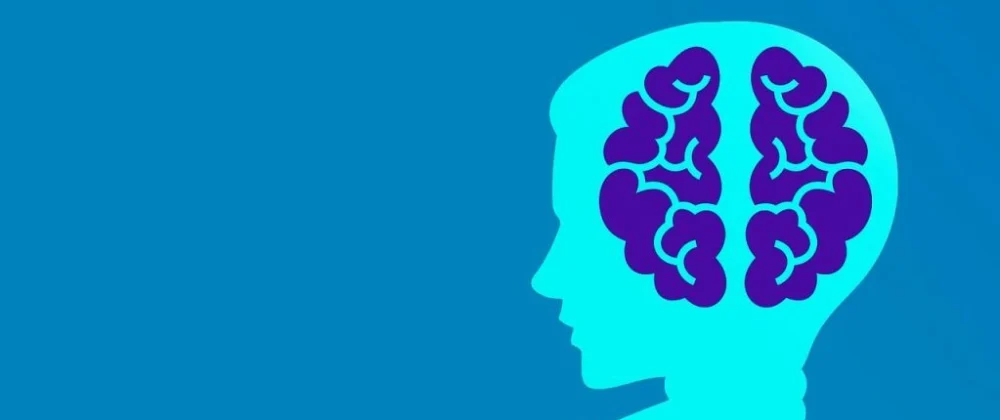
Обработка естественного языка (NLP) — это область искусственного интеллекта, которая позволяет компьютерам понимать, интерпретировать и генерировать человеческий язык.
Он играет решающую роль в современных приложениях, начиная от языкового перевода и анализа настроений и заканчивая чат-ботами и поисковыми системами.
Методы NPL позволяют разработчикам извлекать ценную информацию из огромных объемов текстовых данных, что делает его мощным инструментом для анализа данных и принятия решений.
В первой части этой статьи мы научились настраивать среду и собирать данные, очищать их от нерелевантных слов при подготовке данных для использования в качестве образцов.
В следующем сеансе мы увидим, как использовать данные. Найдите предыдущую сессию здесь.
Теги части речи (POS) в JavaScript
В обработке естественного языка (NLP) тегирование частей речи (POS) является важной задачей, которая включает в себя назначение определенных частей речи, таких как существительные, глаголы, прилагательные и т. Д., Каждому слову в предложении.
Понимание тегов частей речи
Процесс включает в себя анализ каждого слова в предложении и присвоение им соответствующей части речи. Например, в предложении «Быстрая коричневая лиса перепрыгивает через ленивую собаку» слова будут помечены следующим образом:
- "The" -> Определитель (DT)
- "quick" -> Прилагательное (JJ)
- "brown" -> Прилагательное (JJ)
- "fox" -> Существительное (NN)
- "jamps" -> Глагол (VBZ)
- «over» -> Предлог (IN)
- "the" -> Определитель (DT)
- "lazy" -> Прилагательное (JJ)
- "dog" -> Существительное (NN)
Работа с POS-тегами в JavaScript
Мы будем использовать «естественную» библиотеку НЛП; следуйте предыдущему сеансу, чтобы понять, как настроить среду.
Для выполнения тегов POS в JavaScript, который поддерживает различные задачи NLP, включая токенизацию, теги POS и многое другое.
Внедрение POS-тегов
Чтобы реализовать этот шаг, вы должны настроить «естественную» библиотеку, и шаги описаны в первом сеансе этой серии из двух частей. Давайте реализуем POS-теги в JavaScript.
// Import the "natural" library
const natural = require('natural');
// Create a tokenizer and POS tagger instance
const tokenizer = new natural.WordTokenizer();
const posTagger = new natural.BrillPOSTagger(
natural.BrillPOSTagger.defaultRules,
natural.BrillPOSTagger.defaultLexicon
);
// Sample sentence for POS tagging
const sentence = "The quick brown fox jumps over the lazy dog";
// Tokenize the sentence into words
const words = tokenizer.tokenize(sentence);
// Perform POS tagging
const taggedWords = posTagger.tag(words);
// Print the tagged words
taggedWords.forEach((word) => {
console.log(`${word[0]} - ${word[1]}`);
});
Объяснение
- Мы импортируем «естественную» библиотеку и создаем экземпляры для токенизации и маркировки POS, используя
natural.WordTokenizer()
иnatural.BrillPOSTagger()
. - Мы определяем пример предложения, которое мы хотим пометить частями речи.
- Предложение разбивается на отдельные слова с помощью функции
tokenizer.tokenize()
. - Функция
posTagger.tag()
выполняет POS-тегирование токенизированных слов. - Наконец, мы перебираем помеченные слова и печатаем их вместе с соответствующими им частями речи.
Тематическое моделирование в JavaScript
Тематическое моделирование — это метод обучения без учителя, используемый для обнаружения основных тем или тем в наборе текстовых документов. Мы будем использовать образец корпуса документов для извлечения значимых тем.
Понимание тематического моделирования
Тематическое моделирование — это статистический подход, направленный на выявление скрытых тем в большом наборе текстовых документов.
Это позволяет нам определить основные темы или предметы без какой-либо предварительной маркировки или наблюдения со стороны человека.
Одним из популярных алгоритмов тематического моделирования является скрытое распределение Дирихле (LDA).
LDA предполагает, что каждый документ в корпусе представляет собой смесь различных тем, и каждую тему представляет распределение слов.
Затем алгоритм работает итеративно, чтобы присвоить слова различным темам и определить вероятность присутствия каждой темы в данном документе.
К концу процесса мы получаем список тем и слов, которые вносят наибольший вклад в каждую тему.
Работа с тематическим моделированием в JavaScript
Мы будем использовать «естественную» библиотеку NPL для выполнения тематического моделирования в JavaScript. Мы будем использовать образец корпуса документов, чтобы продемонстрировать процесс.
Реализация тематического моделирования
Cover image for Natural Language Processing (NLP) in JavaScript (series 2)
Scofield Idehen
Scofield Idehen
Posted on 19 июл. • Originally published at blog.learnhub.africa
5
1
1
1
1
Natural Language Processing (NLP) in JavaScript (series 2)
#
javascript
#
ai
#
machinelearning
#
nlp
NLP in Javascript (2 Part Series)
1
Natural Language Processing (NLP) in JavaScript (series)
2
Natural Language Processing (NLP) in JavaScript (series 2)
Natural Language Processing (NLP) is a field of artificial intelligence that focuses on enabling computers to understand, interpret, and generate human language.
It plays a critical role in modern applications, ranging from language translation and sentiment analysis to chatbots and search engines.
NLP techniques empower developers to extract insights from vast amounts of textual data, making it a powerful tool for data analysis and decision-making.
In the first part of this article, we learned how to set up an environment and gather data, strip them of irrelevant words while preparing the data to be used as samples.
In the following session, we would see how to use the data. Find the previous session here.
Part-of-Speech (POS) Tagging in JavaScript
In Natural Language Processing (NLP), Part-of-Speech (POS) tagging is a crucial task that involves assigning specific parts of speech, such as nouns, verbs, adjectives, and more, to each word in a sentence.
Understanding Part-of-Speech Tagging
POS tagging plays a vital role in NLP, as it helps in understanding the grammatical structure of a sentence, which is essential for various language-related tasks.
The process involves analyzing each word in a sentence and assigning them their corresponding part of speech. For example, in the sentence "The quick brown fox jumps over the lazy dog," the words would be tagged as follows:
"The" -> Determiner (DT)
"quick" -> Adjective (JJ)
"brown" -> Adjective (JJ)
"fox" -> Noun (NN)
"jumps" -> Verb (VBZ)
"over" -> Preposition (IN)
"the" -> Determiner (DT)
"lazy" -> Adjective (JJ)
"dog" -> Noun (NN)
Working with POS Tagging in JavaScript
We'll use the "natural" NLP library; follow the previous session to understand how to set up your environment.
To perform POS tagging in JavaScript, which supports various NLP tasks, including tokenization, POS tagging, and more.
Implementing POS Tagging
To implement this step, you must set up the "natural" library, and the steps are outlined in the first session of this two-part series. Let's implement POS tagging in JavaScript.
// Import the "natural" library
const natural = require('natural');
// Create a tokenizer and POS tagger instance
const tokenizer = new natural.WordTokenizer();
const posTagger = new natural.BrillPOSTagger(
natural.BrillPOSTagger.defaultRules,
natural.BrillPOSTagger.defaultLexicon
);
// Sample sentence for POS tagging
const sentence = "The quick brown fox jumps over the lazy dog";
// Tokenize the sentence into words
const words = tokenizer.tokenize(sentence);
// Perform POS tagging
const taggedWords = posTagger.tag(words);
// Print the tagged words
taggedWords.forEach((word) => {
console.log(`${word[0]} - ${word[1]}`);
});
Explanation
We import the "natural" library and create instances for tokenization and POS tagging using natural.WordTokenizer() and natural.BrillPOSTagger().
We define a sample sentence we want to tag with parts of speech.
The sentence is tokenized into individual words using the tokenizer.tokenize() function.
The posTagger.tag() function performs POS tagging on the tokenized words.
Finally, we iterate through the tagged words and print them along with their respective parts of speech.
Topic Modeling in JavaScript
Topic modeling is an unsupervised learning technique used to discover underlying themes or topics within a collection of text documents. We'll use a sample corpus of documents to extract meaningful topics.
Understanding Topic Modeling
Topic modeling is a statistical approach aiming to uncover latent topics in a large set of text documents.
It enables us to identify the main themes or subjects without any prior labeling or human supervision.
One of the popular algorithms for topic modeling is Latent Dirichlet Allocation (LDA).
LDA assumes that each document in the corpus is a mixture of various topics, and a distribution of words represents each topic.
The algorithm then works iteratively to assign words to different topics and determine the probability of each topic's presence in a given document.
By the end of the process, we get a list of topics and the words that contribute most to each topic.
Working with Topic Modeling in JavaScript
We will utilize the "natural" NLP library to perform topic modeling in JavaScript. We'll use a sample corpus of documents to demonstrate the process.
Implementing Topic Modeling
// Import the "natural" library
const natural = require('natural');
// Create a new LDA instance
const lda = new natural.LdaSandbox();
// Sample corpus of documents
const documents = [
"Machine learning is an exciting field in computer science.",
"JavaScript is a versatile programming language used for web development.",
"Data science involves extracting insights from data using various techniques.",
"Node.js is a popular runtime environment for server-side JavaScript applications.",
"Topic modeling helps in discovering latent themes from text documents.",
];
// Tokenize the documents
const tokenizer = new natural.WordTokenizer();
const tokenizedDocs = documents.map((doc) => tokenizer.tokenize(doc));
// Perform topic modeling
const numTopics = 2; // Set the number of topics to discover
const numIterations = 1000; // Number of iterations for the algorithm
lda.train(tokenizedDocs, numTopics, numIterations);
// Print the extracted topics
const topics = lda.getTopics();
console.log("Extracted Topics:");
topics.forEach((topic, index) => {
console.log(`Topic ${index + 1}: ${topic.words.join(", ")}`);
});
Explanation
We import the "natural" library, allowing us to work with topic modeling.
A sample corpus of documents is defined, representing the collection of text we want to analyze and extract topics from.
The documents are tokenized into individual words using the natural.WordTokenizer().
We set the number of topics (numTopics) we want the algorithm to discover and the number of iterations (numIterations) for the LDA algorithm to converge.
The lda.train() function performs topic modeling on the tokenized documents.
Finally, we retrieve and print the extracted topics and the most representative words for each topic using the lda.getTopics() function.
Session 8: Text Classification with NLP
Text classification is a vital Natural Language Processing (NLP) task that involves categorizing textual data into predefined classes or categories.
Understanding Text Classification
Text classification is crucial in various real-world applications, including sentiment analysis, spam detection, language identification, and content categorization. The goal is automatically assigning a label or category to a given text document based on its content.
To achieve text classification, we can leverage machine learning algorithms that learn patterns and relationships between the textual data and their corresponding classes.
One commonly used text classification algorithm is the Naive Bayes classifier, which is simple yet effective for many NLP tasks.
Implementing Text Classification
// Import the "natural" library
const natural = require('natural');
// Create a new Naive Bayes classifier instance
const classifier = new natural.BayesClassifier();
// Training data for text classification
const trainingData = [
{ text: "I love this product! It's fantastic.", category: "positive" },
{ text: "This movie was boring and disappointing.", category: "negative" },
{ text: "The weather is lovely today.", category: "positive" },
{ text: "The service at this restaurant was terrible.", category: "negative" },
{ text: "The new software update works perfectly.", category: "positive" },
];
// Training the classifier with the data
trainingData.forEach((data) => {
classifier.addDocument(data.text, data.category);
});
classifier.train();
// Test data for text classification
const testText = "The hotel stay was wonderful! I had a great time.";
// Classify the test data
const predictedCategory = classifier.classify(testText);
// Print the predicted category
console.log(`Predicted Category: ${predictedCategory}`);
Explanation
We import the "natural" library, which provides the necessary tools for text classification.
We create a new instance of the Naive Bayes classifier using natural.BayesClassifier().
The training data contains labeled examples of text and corresponding categories (positive or negative in this case).
The classifier is trained on the training data using the classifier.addDocument() and classifier.train() functions.
We define a test text for which we want to predict the category.
The classifier.classify() function is used to classify the test text into a specific category.
The predicted category is printed on the console.
Language Translation with NLP in JavaScript
Language translation is a crucial NLP application that enables communication and understanding across different languages. This session focuses on language translation techniques and demonstrates how to perform language translation in JavaScript using NLP libraries.
Language Translation Techniques
Language translation can be achieved using different techniques, including rule-based approaches, statistical machine translation, and neural machine translation. In this session, we'll utilize the power of NLP libraries to perform language translation.
Implementing Language Translation in JavaScript
To perform language translation in JavaScript, we can leverage NLP libraries such as "translate-google" and "translate" to access translation services.
Example: Translating Text Using "translate-google" Library
// Import the "translate-google" library
const translate = require('translate-google');
// Text to be translated
const text = "Hello, how are you?";
// Source and target languages
const sourceLanguage = 'en';
const targetLanguage = 'es';
// Translate the text
translate(text, { from: sourceLanguage, to: targetLanguage })
.then((translation) => {
console.log(`Translated Text: ${translation}`);
})
.catch((error) => {
console.error('Translation Error:', error);
});
Example: Translating Text Using "translate" Library
// Import the "translate" library
const translate = require('translate');
// Configure the library with the translation service
translate.engine = 'google';
translate.from = 'en';
translate.to = 'fr';
// Text to be translated
const text = "Good morning, how are you today?";
// Translate the text
translate(text)
.then((translation) => {
console.log(`Translated Text: ${translation}`);
})
.catch((error) => {
console.error('Translation Error:', error);
});
Explanation
We import the required NLP libraries for language translation: "translate-google" or "translate".
We define the text that needs to be translated.
We specify the source language (sourceLanguage) and the target language (targetLanguage) for translation.
The text is translated using the translate() function provided by the respective library.
The translated text is printed to the console.
In the final session of this series, we would look at the use case and future trend of NLP and how its implication in Javascript has the potential to transform learning.
Follow us to see how we build the final project, as this is the first session of a three-part series. If you find this post exciting, find more exciting posts on Learnhub Blog; we write everything tech from Cloud computing to Frontend Dev, Cybersecurity, AI, and Blockchain.
Resource
Getting Started with Programming
Javascript Email with Nodemailer
How to send an email in node.js using nodemailer
Javascript Array method
20 Best React JavaScript Frameworks For 2023
NLP in Javascript (2 Part Series)
1
Natural Language Processing (NLP) in JavaScript (series)
2
Natural Language Processing (NLP) in JavaScript (series 2)
Top comments (0)
Subscribe
pic
Add to the discussion
Code of Conduct • Report abuse
DEV Community
Trending in AI
The AI community is currently focused on the use of GPT in generating full-stack web apps and improving coding workflows, while also discussing the potential drawbacks of over-reliance on AI tools like GitHub Copilot.
Wasp
How we built a GPT code agent 🤖 that generates full-stack web apps in React & Node.js, explained simply
Martin Šošić for Wasp ・ Jul 18
#chatgpt #react #webdev #ai
GitHub
How I'm using GitHub Copilot to learn p5.js
Rizèl Scarlett for GitHub ・ Jul 10
#ai #githubcopilot #learning #programming
renanfranca
💨🚀 Accelerate Your Workflow with ChatGPT Prompts 📝 - Ditch the Boilerplate 📃✖️ and Eliminate Duplicates 🔄🚫
Renan Franca ・ Jul 12
#ai #beginners #javascript #tutorial
wynandpieters
ChatGPT has made me more productive (but at what cost?)
Wynand Pieters ・ Jul 14
#ai #coding #productivity #ethics
mitchiemt11
Is the AI Hype Over? OpenAI's ChatGPT Code Interpreter Takes Center Stage
Mitchell Mutandah ・ Jul 9
#ai #discuss #news
Read next
sumukhakb210 profile image
Creating an Animated and Accessible Accordion with JavaScript, HTML, and CSS
Sumukhakb - Jun 27
ajmal_hasan profile image
React-Native-MMKV storage
Ajmal Hasan - Jul 8
qbentil profile image
Increasing productivity: Best practices for React beginners.
Bentil Shadrack - Jul 5
alvaromontoro profile image
10 Cool CodePen Demos (June 2023)
Alvaro Montoro - Jul 5
Scofield Idehen
Follow
I was lost, but tech found me...
LOCATION
World
EDUCATION
University of Benin
WORK
LinkOrion Technology
JOINED
23 мар. 2022 г.
More from Scofield Idehen
Natural Language Processing (NLP) in JavaScript (series)
#javascript #machinelearning #nlp #ai
JavaScript and its many Framework
#javascript #webdev #programming #beginners
Understanding DOM Manipulation with JavaScript
#webdev #javascript #react #programming
DEV Community
OpenAI
Ready to explore the possibilities of ChatGPT?
Create a DEV account and follow tags such as #openai, #gpt3, #chatgpt, and more
Discover the latest advancements in AI technology.
New content every day.
// Import the "natural" library
const natural = require('natural');
// Create a new LDA instance
const lda = new natural.LdaSandbox();
// Sample corpus of documents
const documents = [
"Machine learning is an exciting field in computer science.",
"JavaScript is a versatile programming language used for web development.",
"Data science involves extracting insights from data using various techniques.",
"Node.js is a popular runtime environment for server-side JavaScript applications.",
"Topic modeling helps in discovering latent themes from text documents.",
];
// Tokenize the documents
const tokenizer = new natural.WordTokenizer();
const tokenizedDocs = documents.map((doc) => tokenizer.tokenize(doc));
// Perform topic modeling
const numTopics = 2; // Set the number of topics to discover
const numIterations = 1000; // Number of iterations for the algorithm
lda.train(tokenizedDocs, numTopics, numIterations);
// Print the extracted topics
const topics = lda.getTopics();
console.log("Extracted Topics:");
topics.forEach((topic, index) => {
console.log(`Topic ${index + 1}: ${topic.words.join(", ")}`);
});
Объяснение
- Мы импортируем «естественную» библиотеку, позволяющую нам работать с тематическим моделированием.
- Определен примерный корпус документов, представляющий набор текстов, которые мы хотим проанализировать и извлечь из них темы.
- Документы разбиваются на отдельные слова с помощью функции
natural.WordTokenizer()
. - Мы устанавливаем количество тем (numTopics), которые мы хотим, чтобы алгоритм обнаружил, и количество итераций (numIterations) для сходимости алгоритма LDA.
- Функция
lda.train()
выполняет тематическое моделирование токенизированных документов. - Наконец, мы извлекаем и печатаем извлеченные темы и наиболее репрезентативные слова для каждой темы, используя функцию
lda.getTopics()
.
Сессия 8: Классификация текстов с помощью NPL
Классификация текста является жизненно важной задачей обработки естественного языка (NLP), которая включает в себя классификацию текстовых данных по заранее определенным классам или категориям.
Понимание классификации текста
Классификация текста имеет решающее значение в различных реальных приложениях, включая анализ настроений, обнаружение спама, идентификацию языка и категоризацию контента. Целью является автоматическое присвоение метки или категории данному текстовому документу на основе его содержимого.
Чтобы добиться классификации текста, мы можем использовать алгоритмы машинного обучения, которые изучают шаблоны и отношения между текстовыми данными и соответствующими им классами.
Одним из часто используемых алгоритмов классификации текста является наивный байесовский классификатор, который прост, но эффективен для многих задач NPL.
Реализация текстовой классификации
// Import the "natural" library
const natural = require('natural');
// Create a new Naive Bayes classifier instance
const classifier = new natural.BayesClassifier();
// Training data for text classification
const trainingData = [
{ text: "I love this product! It's fantastic.", category: "positive" },
{ text: "This movie was boring and disappointing.", category: "negative" },
{ text: "The weather is lovely today.", category: "positive" },
{ text: "The service at this restaurant was terrible.", category: "negative" },
{ text: "The new software update works perfectly.", category: "positive" },
];
// Training the classifier with the data
trainingData.forEach((data) => {
classifier.addDocument(data.text, data.category);
});
classifier.train();
// Test data for text classification
const testText = "The hotel stay was wonderful! I had a great time.";
// Classify the test data
const predictedCategory = classifier.classify(testText);
// Print the predicted category
console.log(`Predicted Category: ${predictedCategory}`);
Объяснение
- Мы импортируем «естественную» библиотеку, которая предоставляет необходимые инструменты для классификации текста.
- Мы создаем новый экземпляр наивного байесовского классификатора, используя
natural.BayesClassifier()
. - Данные обучения содержат помеченные примеры текста и соответствующие категории (в данном случае положительные или отрицательные).
- Классификатор обучается на обучающих данных с использованием функций
classifier.addDocument()
иclassifier.train()
. - Мы определяем тестовый текст, для которого мы хотим предсказать категорию.
- Функция
classifier.classify()
используется для классификации тестового текста в определенную категорию. - Прогнозируемая категория печатается на консоли.
Языковой перевод с помощью NPL в JavaScript
Языковой перевод является важным приложением НЛП, которое обеспечивает общение и понимание на разных языках. На этом занятии основное внимание уделяется методам языкового перевода и демонстрируется, как выполнять языковой перевод в JavaScript с использованием библиотек НЛП.
Методы языкового перевода
Языковой перевод может быть достигнут с использованием различных методов, включая подходы, основанные на правилах, статистический машинный перевод и нейронный машинный перевод. На этом занятии мы будем использовать возможности библиотек НЛП для выполнения языкового перевода.
Реализация языкового перевода в JavaScript
Чтобы выполнить языковой перевод в JavaScript, мы можем использовать библиотеки NLP, такие как «translate-google» и «translate», для доступа к службам перевода.
Пример: перевод текста с помощью библиотеки «translate-google»
// Import the "translate-google" library
const translate = require('translate-google');
// Text to be translated
const text = "Hello, how are you?";
// Source and target languages
const sourceLanguage = 'en';
const targetLanguage = 'es';
// Translate the text
translate(text, { from: sourceLanguage, to: targetLanguage })
.then((translation) => {
console.log(`Translated Text: ${translation}`);
})
.catch((error) => {
console.error('Translation Error:', error);
});
Пример: перевод текста с помощью библиотеки «translate»
// Import the "translate" library
const translate = require('translate');
// Configure the library with the translation service
translate.engine = 'google';
translate.from = 'en';
translate.to = 'fr';
// Text to be translated
const text = "Good morning, how are you today?";
// Translate the text
translate(text)
.then((translation) => {
console.log(`Translated Text: ${translation}`);
})
.catch((error) => {
console.error('Translation Error:', error);
});
Объяснение
- Импортируем необходимые библиотеки НЛП для языкового перевода: «translate-google» или «translate».
- Определяем текст, который необходимо перевести.
- Мы указываем исходный язык (sourceLanguage) и целевой язык (targetLanguage) для перевода.
- Текст переводится с помощью функции translate(), предоставляемой соответствующей библиотекой.
- Переведенный текст выводится на консоль.
На заключительном занятии этой серии мы рассмотрим варианты использования и будущие тенденции НЛП, а также то, как его применение в Javascript может изменить обучение.